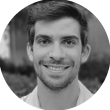
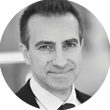
Development and Validation of a Pixel Wise Deep Learning Model to Detect Cataract on Swept-Source Optical Coherence Tomography Images
Zéboulon P, Panthier C, Rouger H, Bijon J, Ghazal W, Gatinel D1
Industry support: None
ABSTRACT SUMMARY
Investigators sought to develop and validate a deep learning model to detect and localize cataract on swept-source anterior segment OCT (AS-OCT) scans. The deep learning model was trained on 504 swept-source OCT (SS-OCT) images from patients with either clear crystalline lenses or cataracts. The images were annotated pixel-wise. Clear lens pixels were labeled entirely as normal, and cataractous lenses were labeled entirely as cataract. The model's performance was validated on 1,326 different images of 114 patients. It produced a pixel-wise probabilistic heatmap indicating the likelihood of cataract on each pixel of an SS-OCT image. The results showed an area under the receiver operating characteristic curve of 0.98 and a sensitivity and specificity for detecting cataract of 94.4% and 94.7%, respectively.
Study in Brief
Investigators developed and validated a deep learning model for detecting and localizing cataract on swept-source anterior segment OCT images. The model produced a pixel-wise probabilistic heatmap indicating the likelihood of cataract on each pixel of a swept-source OCT image.
WHY IT MATTERS
The study showed that anterior segment OCT could be a valuable tool for the diagnosis, monitoring, and treatment of cataracts. Compared to traditional slit-lamp biomicroscopy, the technique was more objective, repeatable, and operator independent. The adoption of a pixel-wise detection approach could allow a more thorough comprehensive assessment of disease compared to the conventional image classification algorithm.
DISCUSSION
There is a growing demand for tools and devices that are objective, repeatable, and more operator independent. AS-OCT has become increasingly popular in anterior segment screening and diagnosis. Additionally, Zéboulon et al developed a deep learning model for detecting and localizing cataract on SS-OCT images.
The 1,300-nm wavelength of the Anterion (Heidelberg Engineering), the AS-OCT device used in the study, enables deeper penetration and visualization of the crystalline lens than its 800-nm counterpart.2 These advantages may come at the expense of lower axial resolution but are particularly useful in the diagnosis and treatment of cataracts.3 Unlike slit-lamp biomicroscopy, AS-OCT does not have a variable light beam intensity, and the technology can isolate the lens and eliminate other confounding factors to the examination such as corneal and vitreous opacities.
In many cases, cataract presents as a heterogenous disease. The study’s use of a pixel-wise detection method rather than a traditional image classification algorithm allowed a more comprehensive and accurate assessment of the type of cataract that was developing, including early cataracts that had yet to affect vision.
Overall, the study found that the tested deep learning model could provide valuable assistance to physicians in the diagnosis, monitoring, and treatment of cataracts.
PhacoTrainer: A Multicenter Study of Deep Learning for Activity Recognition in Cataract Surgical Videos
Yeh HH, Jain AM, Fox O, Wang SY4
Industry support: None
ABSTRACT SUMMARY
Investigators attempted to build and evaluate deep learning models for recognizing cataract surgical steps from full-length surgical videos. A total of 298 cataract surgical videos from 12 resident surgeons across six sites were collected, and 13 surgical steps were labeled in each video. Two deep learning models were trained, one based on the Visual Geometry Group 16 architecture (VGG model), which is a convolutional neural network (CNN) architecture for image-based deep learning, and the other using VGG16 followed by a recurrent neural network (CNN-RNN model), a deep learning architecture that makes sense of sequential data. The results showed a prediction accuracy of 76% and 84% for the VGG and CNN-RNN models, respectively. The microaveraged area under receiver operating characteristic curves was 0.97 and 0.99 for the VGG and CNN-RNN models, respectively.
Study in Brief
Investigators aimed to build and evaluate deep learning models for recognizing cataract surgical steps in full-length surgical videos. Two deep learning models were trained and tested on 298 cataract surgical videos collected from 12 resident surgeons across six sites. The results showed high accuracy in recognizing the 13 surgical steps.
WHY IT MATTERS
The ability of deep learning models to recognize cataract surgical steps accurately from full-length surgical videos may have significant implications for surgical training and quality control. Automated feedback metrics such as the length of time spent on each step could provide valuable information for resident surgeons.
DISCUSSION
The study demonstrated the capability of deep learning models to classify cataract surgical activity on a frame-by-frame basis with remarkable accuracy.
For an ophthalmology resident in training, cataract surgery feedback is mostly verbal and limited to the OR. Recognizing cataract surgical steps with AI could improve surgical training by analyzing surgical videos and computing relevant performance metrics such as time spent on each step or the number of operation steps. Both metrics are correlated with surgeon performance; less experienced surgeons take more time, on average, during a surgery and complete more operative steps owing to repeats.5
The study is a stepping stone to the implementation of context-aware computer-assisted or even fully robotic surgery.6,7 Such systems have the potential to perform real-time adaptation of device settings, deliver valuable insight, and provide real-time feedback or danger notifications to surgeons.
1. Zéboulon P, Panthier C, Rouger H, Bijon J, Ghazal W, Gatinel D. Development and validation of a pixel wise deep learning model to detect cataract on swept-source optical coherence tomography images. J Optom. 2022;15(suppl 1):S43-S49.
2. Xie X, Corradetti G, Song A, et al. Age- and refraction-related changes in anterior segment anatomical structures measured by swept-source anterior segment OCT. PLoS One. 2020;15(10):e0240110.
3. Aumann S, Donner S, Fischer J, Müller F. Optical coherence tomography (OCT): principle and technical realization. In: Bille JF, ed. High Resolution Imaging in Microscopy and Ophthalmology: New Frontiers in Biomedical Optics. Springer; 2019:59-85.
4. Yeh HH, Jain AM, Fox O, Wang SY. PhacoTrainer: a multicenter study of deep learning for activity recognition in cataract surgical videos. Transl Vis Sci Technol. 2021;10(13):23.
5. Schoeffmann K, Taschwer M, Sarny S, Münzer B, Primus MJ, Putzgruber D. Cataract-101: video dataset of 101 cataract surgeries. MMSys ’18: Proceedings of the 9th ACM Multimedia Systems Conference. 2018:421-425.
6. Franke S, Rockstroh M, Hofer M, Neumuth T. The intelligent OR: design and validation of a context-aware surgical working environment. Int J Comput Assist Radiol Surg. 2018;13(8):1301-1308.
7. Kitaguchi D, Takeshita N, Matsuzaki H, et al. Real-time automatic surgical phase recognition in laparoscopic sigmoidectomy using the convolutional neural network-based deep learning approach. Surg Endosc. 2020;34(11):4924-4931.