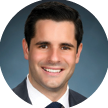
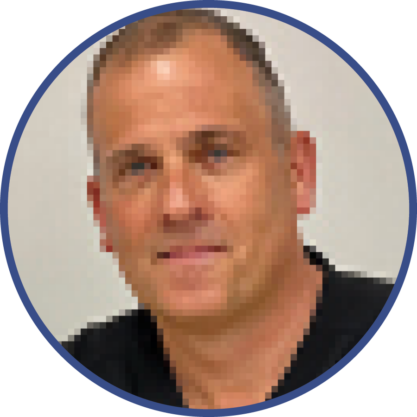
IOL calculation formulas have been static for the past 30 years. Even newer-generation formulas with effective lens position determination and increased input parameters such as white-to-white distance, anterior chamber depth, and lens thickness (eg, Barrett Universal, Holladay 2) are limited because the parameters are fixed in terms of quantity and type.
The only way for surgeons to improve their refractive results with static formulas is via A-constant optimization. This process is akin to shifting a function up and down on the y-axis by changing the y-intercept (Figure 1). It has inherent limitations because it treats all eyes equally, including those at the biometric extremes. For example, a small eye with a steep cornea is treated with the same fudge factor as a long eye with a deep anterior chamber.
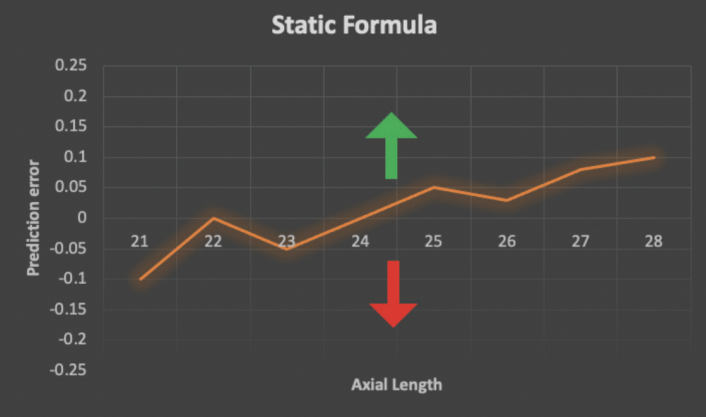
Figure 1. The shape of a static formula cannot be optimized with new outcomes data. The only way to modify such a formula is via A-constant optimization, a process that shifts the entire formula contour up (green arrow) or down (red arrow).
Modern formulas that leverage machine learning, also known as AI formulas, are theoretically more accurate for three reasons. First, they are created using large datasets. Second, they use advanced machine learning algorithms that are excellent at creating predictive models. Third, they can be updated and improved as postoperative refractive data become available (Figure 2). Examples of publicly available AI formulas include the Radial Basis Function (RBF) 2.0, Emmetropia Verifying Optical Formula (EVO) 2.0, Kane, Prediction Enhanced by Artificial Intelligence and Output Linearization–Debellemaniére, Gatinel, Saad (PEARL-DGS), Hoffer Q Savini/Taroni (Hoffer QST), and Ladas Super 2.0. None, however, has been reported to achieve greater than 90% accuracy for a prediction error within ±0.50 D.1 The Precision Ladas Universal Super (PLUS) algorithm has been developed in an effort to move the needle.
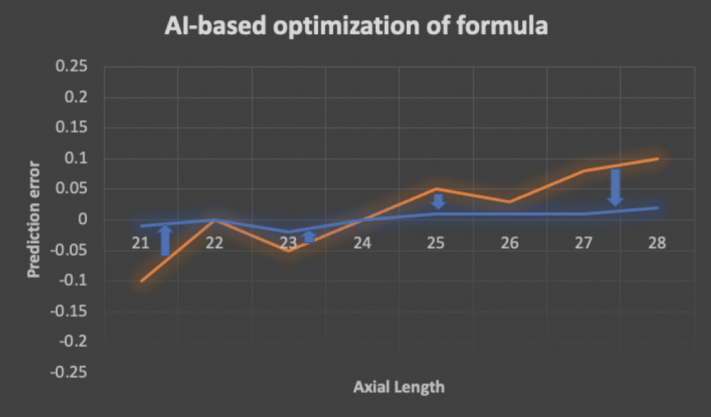
Figure 2. An AI-based formula can be modified and improved as postoperative data are fed into the model. Prediction errors are minimized (blue arrows) as the original starting formula (orange line) is transformed into an updated formula (blue line).
IMPROVING ACCURACY AND EFFICIENCY
Roadblocks. We believe there are two major roadblocks to achieving greater accuracy benchmarks. The first is an inability to acquire large amounts of high-quality postoperative refractive data for a specific surgeon or group of surgeons. The second is the difficulty of feeding postoperative data back into an AI model—usually via manual entry on online websites—to train, retrain, and update it.
A potential solution. Our goal with PLUS is to achieve greater than 90% accuracy and improve virtually any surgeon’s results with a training set of only a few hundred eyes. The most state-of-the-art AI algorithms (eg, ensemble learning with gradient boosting) are applied to the model to focus on the validation and quality control of postoperative refractive data and improve workflow efficiencies related to acquiring data and updating the model.
The PLUS model can be customized for a particular surgeon, or it can be generalized for many surgeons by pooling data.
Customized. In a recent analysis (September 2022), we obtained data from three expert surgeons and were able to improve their outcomes with their preferred formulas by using outcomes data from as few as 500 eyes and our PLUS methodology. We have consistently demonstrated improvements over a surgeon’s baseline to as high as 94% accuracy.
Pooled data. Alternatively, PLUS can pool and apply internal data from various companies with any baseline formula to improve results for surgeons.
DATA INTEGRATION
Our vision for PLUS, as for AI-based models in general, is to integrate it with the following:
- The software and hardware necessary for cataract surgery planning;
- Electronic health record systems where the specifications of the IOL implanted and postoperative manifest refraction data can be accessed; and
- A wavefront analyzer/autorefractor where objective postoperative data can be acquired (patent pending).
A cloud-based neural net model would be used first to cross-validate the postoperative manifest refraction with postoperative autorefraction/wavefront analyzer data and then to update the model automatically if the input data meet certain high-quality standards (Figure 3).
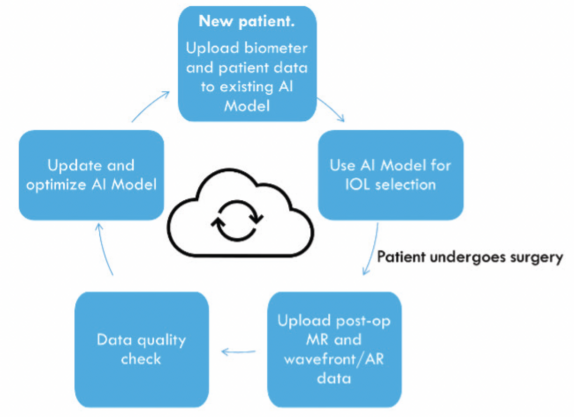
Figure 3. The authors’ vision of an AI-based IOL selection model using a cloud-based workflow (patent pending).
ADVANTAGES
The following are four possible advantages of PLUS:
No. 1. The model’s ability to improve and retrain itself in perpetuity;
No. 2. The ability to add input parameters (eg, age or total keratometry values) to the model as they become available without having to create a model from scratch;
No. 3. The ability to apply methodology to specific patient populations (eg, individuals with a history of myopic LASIK or Fuchs dystrophy); and
No. 4. The ability to apply methodology to other areas of refractive surgery such as LASIK (patent pending), ophthalmic specialties, or data-driven areas of medicine in which numerical predictions can be compared against results or gold standards.
CONCLUSION
(Authors’ note: This conclusion was partially generated by entering the following prompt into ChatGPT Plus: “Please write a concluding paragraph for an essay entitled, ‘The Future of AI in IOL Power Calculations.’”)
The use of AI in IOL power calculations holds tremendous potential for innovation and growth. Advances and integration with other technologies could improve the accuracy and personalization of cataract surgery and give surgeons greater confidence in IOL planning. Fostering ongoing research and development in AI-based solutions and collaboration between scientists, practitioners, and industry could position cataract surgery at the forefront of precision medicine and patient care.
1. Taroni L, Hoffer KJ, Barboni P, Schiano-Lomoriello D, Savini G. Outcomes of IOL power calculation using measurements by a rotating Scheimpflug camera combined with partial coherence interferometry. J Cataract Refract Surg. 2020;46(12):1618-1623.